A Transformative Cure: How AI Can Change Healthcare
The healthcare sector is swimming in data—text, images, videos, and more—following the digitization of the last decades. We believe this wealth of data will allow artificial intelligence (AI) to transform healthcare and life sciences. AI can derive insights from data in ways that transcend human capabilities—providing potentially unprecedented understanding of complex biology and physiology. It has the potential to speed up drug development at lower costs and improve the effectiveness of new medicines.
Amit Sinha, Global Head of Life Sciences at Goldman Sachs, recently interviewed Anima Anandkumar, a Bren Professor of Computing and Mathematical Sciences at the California Institute of Technology and Senior Director of AI Research at Nvidia, about what AI means for life sciences innovation.
Your research has focused on several different fields, from weather prediction to drug discovery to robotics. Which sectors could be most impacted by AI in the coming decade?
We’re just at the beginning of a really exciting revolution that’s going to impact all kinds of fields—we’ve all seen that AI can generate new text and new images, but it also has scope to generate new proteins, new drugs, new car designs, better medical instruments. Everything now seems possible.
One area that AI is transforming is precision medicine—the idea you can use it to design better drugs and then get those better drugs to the right patients. Can you explain how that works?
Since the 17th century, people have been saying, “I'm going to go try this experiment,” and whether it’s on mice or humans, it takes take a long time. If it succeeds, that’s great. If it fails, you might not even know why and it’s back to the drawing board.
But what if we could make it much more systematic and explore, say, drug design in greater depth than before? AI can ultimately bring all those physical experiments into the virtual world. The real world is so complex. But if you can make more and more accurate predictions in simulations, and speed up the ability to simulate, we can answer questions such as how well a drug binds to targets in the human body more quickly and accurately.
Research becomes more cost-effective and much faster by making it more computational and reducing our reliance on physical experiments. But we still need the physical experiments in the end to validate our results.
The cost of genomic sequencing is falling, so we’re seeing targeting of drugs improve. Once we can identify the differences between certain populations at the genomic level, it’s possible to target drugs better. It’s vital that we gather data at the human population level so we can feed it into our AI models. This is about considering the specifics of a drug, taking a dataset around population or genetics, and then mapping them together to see who's going to be best suited to benefit from that drug. All this is in the realm of big data, and that's where AI shines.
What does AI mean for repurposing existing drugs for new applications?
To me, drug repurposing is great. It’s relatively low-hanging fruit in that we already have a database of information about existing drugs, their safety profile and what they target. Lots of the research has already been conducted. Take a drug that's approved for one indication and through AI-enabled research, you can repurpose that drug and you may find that it has usefulness in another disease. That means it's much easier and cheaper than asking AI to generate a new candidate molecule from scratch.
How can AI help to improve early disease detection and predictions?
Everyone knows that prevention is better than cure, but until now that hasn't really been feasible other than advocating some lifestyle changes. But now it can be data-driven. Apple Watch,1 for instance, is taking regular measurements and can use that data to identify heart irregularities, possibly helping with early detection of heart attacks.
One of my colleagues works on sweat sensors. They can provide information about diabetes or other conditions just using your sweat. There are other sensors out there that are cheap, broadly accessible, low powered and can use AI to detect abnormalities and make recommendations or call for help if there’s an adverse event.
AI is also helping detect tumors early and in a non-invasive way. For example, it’s making full-body scans much faster and cheaper and helping reconstruct much higher-resolution images without requiring hours of computing. Big data also has a role to play. We can look at data at the broad demographic level and see patterns emerge that can help us determine what, for example, the early warning signs for heart disease are.
From a technology perspective, what’s still needed to drive innovation? Do firms even have access to the required computing power in terms of chips and the cloud?
AI is really a trinity of data, algorithms, and computer infrastructure. The data side of it in some domains has been readily accessible. For instance, text and images are freely available on the internet, and that's why we’re seeing the fastest innovations there. But in other areas like life sciences, it's slower because the data is fragmented and not at the scale needed for patterns to emerge.
AI is making it thousands of times faster to develop surrogates and better simulations. That’s purely computing power involved—there’s no data. But sometimes data is the limiting factor—do you have enough genomes to build a large language model? That’s exactly what we did last year. We took all the known virus and bacterial genomes and trained a language model. But instead of using English text, it's trained on nucleotides—the chemicals that make up the genetic code. Using that, we can predict new variants of concern for coronavirus and other infectious diseases before they even emerge.
Sometimes when we don’t have enough data, like for human trials of drugs when there’s only ever a limited amount, we need to try and make sense of the data we have. In these cases, we need better algorithmic techniques.
We’ve recently seen partnerships between technology companies and biotechnology companies. Is this the future of innovation in life sciences?
It’s a really exciting development. These tech companies have huge expertise in building large computer clusters and developing software and AI frameworks that help us train on big data. We can’t expect life sciences companies to hire all the talent they’d need to replicate these capabilities.
It’s no longer a case of one person sitting at a desktop, using a small dataset, and running some machine learning that is available open source to come up with some innovations. The cutting edge of life sciences now involves big data, building large language models and huge computing power. You need specialist expertise to be able to engineer and build these models.
What do you recommend to life sciences companies looking to incorporate AI and machine-learning technology into their core innovation processes?
I’d say not to just put AI in a pitch deck just because it's glamorous at the moment. That might excite some people, but not the investors who really understand what’s going on and what you’re doing. If a company uses AI, it needs to be integrated in its processes properly. But beforehand, consider whether AI is even the right thing for the company. In some cases, maybe it's not.
1 For illustrative purposes only. Any reference to a specific company or security does not constitute a recommendation to buy, sell, hold or directly invest in the company or its securities.
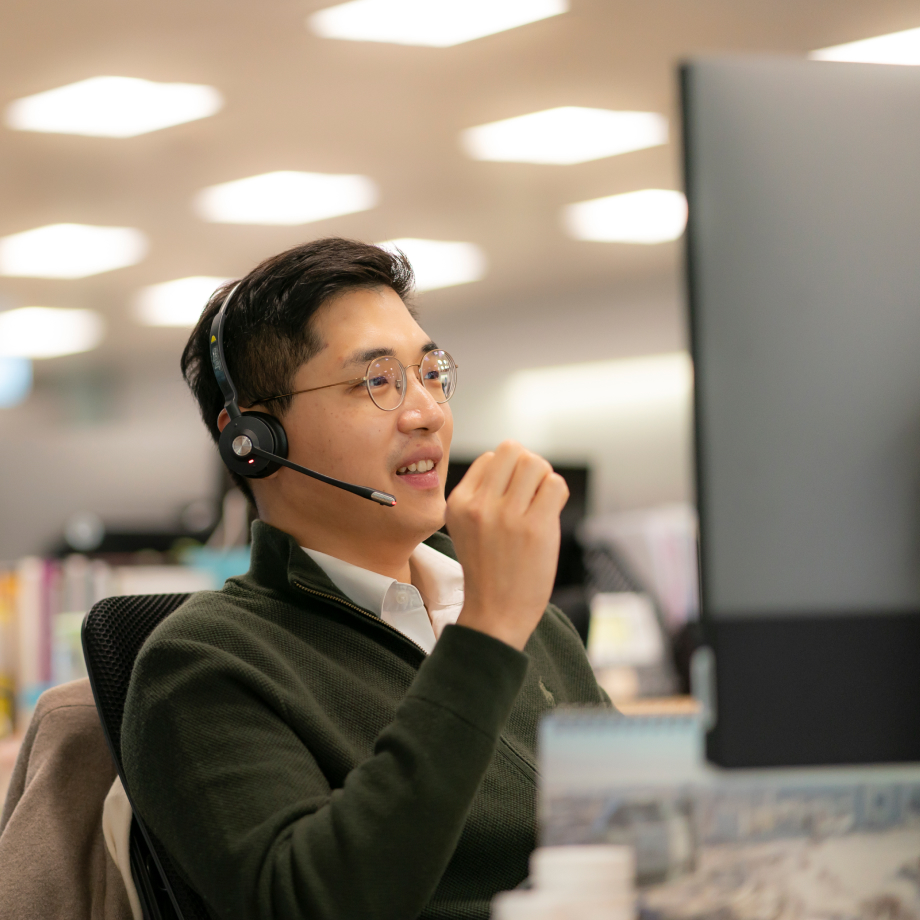