Artificial Intelligence: Data is the Differentiator
The rapid rise and transformative potential of generative AI (GenAI) puts it on course to become a hallmark of the modern economy. We expect the range of investment opportunities and risks to expand as AI alters economies and industries, with the dispersion between winners and losers widening. We’ve previously highlighted that potential beneficiaries can be found beyond a concentrated group of large US companies that have so far led the equity market higher. First order beneficiaries—what we call the “enablers”—including semiconductor manufacturers. We think the next set of potential beneficiaries can be found in the “data and security” layer.
The amount of data being created is growing exponentially. Datasets are also becoming larger, more unstructured, and complex. This creates an ideal environment for the development of innovative AI technologies, such as large language models. However, studies suggest a top challenge preventing companies from scaling their AI ambitions is data management—from collection and storage, to cleaning and protection.1 We believe companies across the tech ecosystem that can help businesses with these tasks are set to disproportionately benefit as we move further into an AI world.
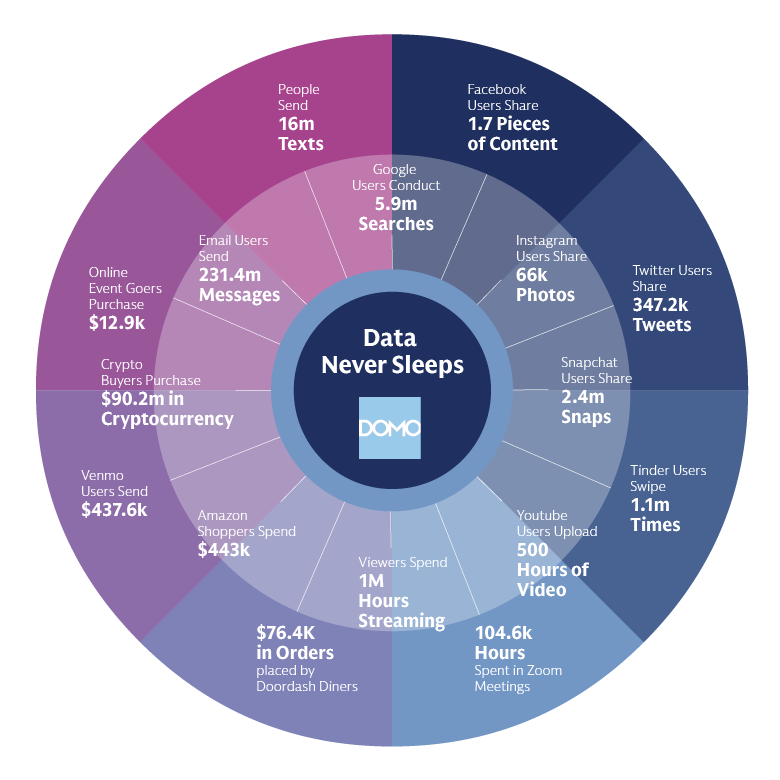
Source: Domo, originally released in September 2022 and subsequently updated. Any reference to a specific company or security does not constitute a recommendation to buy, sell, hold or directly invest in the company or its securities.
From Collection to Protection
Collection
The advent of commercially accessible large language models, such as ChatGPT, require significant computational power and memory. But ultimately, they run on data. Throughout the training phase, large language models detect patterns and relationships within the inputted data. The model then predicts outcomes. To best prepare a model for its desired scale and scope of use cases, a wide range of relevant, accurate, and comprehensive data is essential. Yet CEOs are concerned that data lineage and provenance will be a barrier to adopting GenAI.2
Data falls into one of two categories: structured or unstructured. In its raw form, structured data is quantitative and, therefore, organized. Unstructured data is qualitative—such as audio, video, social media posts—and requires additional effort to interpret. While structured data is easier to decipher, unstructured data provides a deeper understanding into habits and behavior when analyzed effectively, thereby delivering more meaningful results, and driving enhanced outcomes.
To train models to their full potential, data engineers collect a combination of structured and unstructured data for a model to ingest. Ultimately, the range of outputs are determined by the patterns learned by a given model based on the inputs. The effectiveness of an AI model is limited by the training data, underscoring the dependence on representative, high-quality, and accurate datasets. We believe companies that lean into unstructured data will create amplified efficiencies and deliver better results.
Storage
Once collected, data storage options range from on-premise, public or private cloud environments, and hybrid solutions. Each option has its own benefits and limitations.
- On-premise storage offers autonomy and control over the hardware, which might be preferred when dealing with sensitive data and/or regulatory considerations. A large upfront capital investment is required for on-premise storage and scalability is limited.
- Cloud environments provide scalability, accessibility, and generally operate under a usage-based cost structure. However, the dependency on external service providers could create data privacy concerns.
- Hybrid solutions combine the benefits of on-premise and cloud environments. While a hybrid approach offers a high degree of flexibility regarding scale, cost, and efficiency, it also requires complex integration and expertise.
In recent years, there has been a large shift toward cloud environments. In addition to being catalyzed by the pandemic, increased momentum in AI workloads and AI-related services is set to contribute to cloud computing growth and margins going forward. We expect companies within the cloud ecosystem stand to benefit, including network infrastructure providers facilitating the transition from on-premise to cloud platforms.
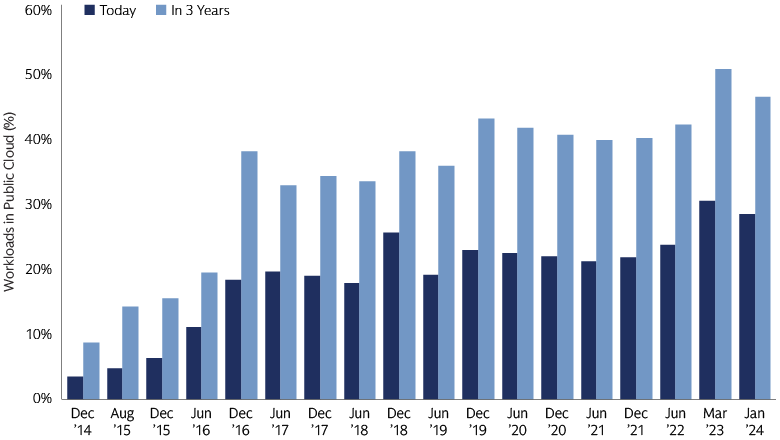
Source: Goldman Sachs Global Investment Research. As of March 18, 2024. 100 IT executives surveyed from Global 2000 companies. Question: "What percent of your applications have you moved to public cloud platforms today and what do you expect in 3 years from now (e.g., Amazon AWS, Microsoft Azure, Google Cloud)?" Any reference to a specific company or security does not constitute a recommendation to buy, sell, hold or directly invest in the company or its securities.
Cleaning
It is key for companies to have confidence the output of AI models. Model predictability—understanding the data lineage and accuracy—is integral to earning the trust of users and stakeholders. We expect corporate decision makers to leverage AI for important choices, ranging from credit ratings to healthcare decisions. High-stake scenarios emphasize the necessity for high-quality data within the context of AI models.
When feeding data into AI models, unorganized and inaccurate data can be expensive and detrimental to the model’s performance due to costly errors and time spent on corrections. Data cleaning services help maintain integrity within the data value chain and satisfy data lineage traceability requirements. We believe there is a significant opportunity to invest in companies that offer services to prepare and clean data.
Protection
The protection of data for training AI models is paramount in a world where cyberattack methods are constantly evolving as hackers find and exploit new vulnerabilities. The rise in volume and sophistication of cyberattacks is being driven by increased digitization globally and the complex geopolitical environment. AI’s advancing capabilities may also be used to develop cyberattacks and enable data breaches, but they will also be a critical component of successful defense, potentially identifying hidden activity and complex patterns of malicious behavior.
As the threat landscape evolves, corporate decision makers responsible for information security will require new solutions to secure their company’s proprietary data. Next generation cybersecurity providers can help clients protect their most valuable asset. In the years ahead, we expect innovation and market expansion to drive investment opportunities in robust cyber defense technologies.
The Power is in the Data
As AI models become more sophisticated and complex, a company’s limit within the context of GenAI capabilities will be determined by the quality of its data and ability to manage it effectively throughout its lifecycle. This includes where the data originates, storage infrastructure, cleansing protocols, and measures in place to keep it secure.
We seek to identify companies that are enabling businesses to effectively manage their data with discipline rather than pollute their AI ecosystems with insufficient or inaccurate data. As active investors, we scrutinize potential opportunities to invest in companies that are leveraging data to enhance operational efficiency, better understand their customers, create more personalized experiences, and make more informed decisions driven by the application of AI. We believe these companies will begin to differentiate themselves in 2024 and beyond as AI technologies mature and the investment opportunity set widens.
1 McKinsey. The data dividend: Fueling generative AI. As of September 15, 2023.
2 IBM Institute for Business Value. Generative AI CEO pulse survey. 200 US CEOs. As of April/May 2023.
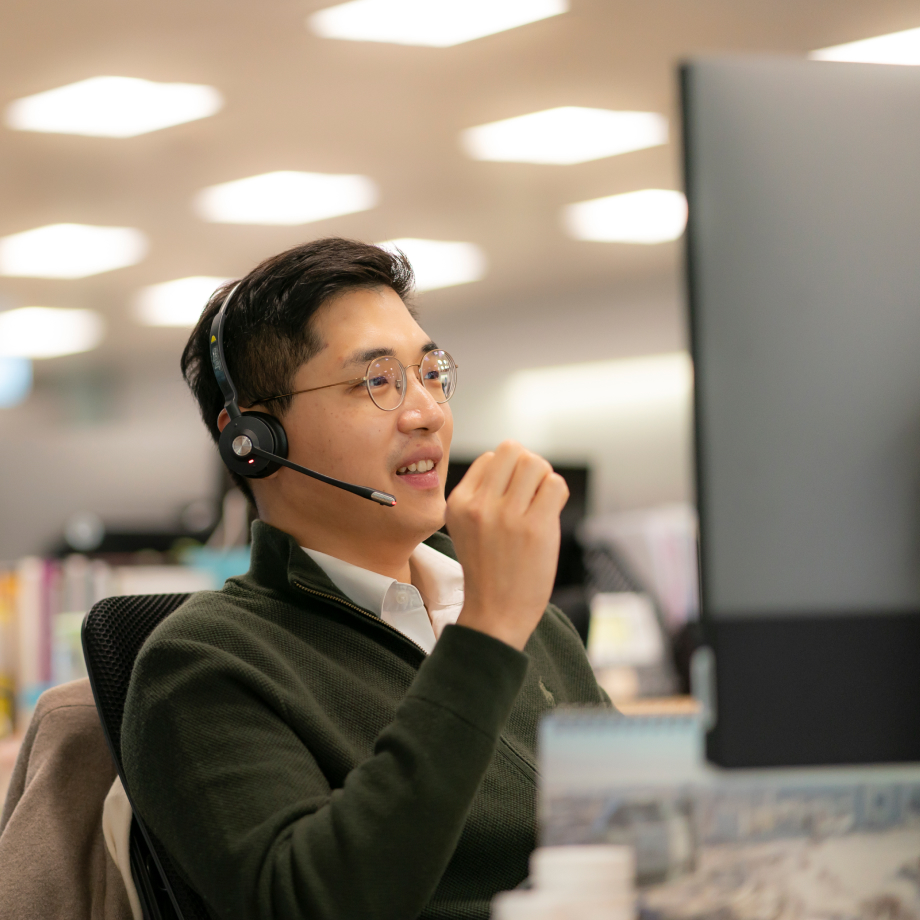