A Framework for Companies to Effectively Deploy AI-Generative AI
The Value Accelerator team has developed a three-stage framework for companies to leverage when deploying Generative Artificial Intelligence (GenAI).
Approaches to AI/GenAI have begun to mature over the last 12 months as companies embark on pilots and extend their learnings to the broader organization. The Goldman Sachs Value Accelerator (VA) team has been advising many of our portfolio companies on over 20 GenAI pilots they are developing for their businesses and scaled up use cases in this same timeframe.
Most of the AI use cases at GS portfolio companies to date can be captured in the following 4 categories:
- Knowledge Management: Boost productivity and employee experience by allowing quick information extraction from disparate sources (with minimal questions)
- Customer Experience: Enhance customer experience by better understanding customer needs and proactively addressing calls or queries through AI generated (and company data informed) response in a fraction of the time
- Coding and Developer Productivity: Improve coding and increase developer efficiency through auto-generating code functions, standardizing code documentation, and rapidly adapting code from existing libraries
- Content Generation: Accelerate content generation by leveraging the multi-modal capabilities of Large Language Models (LLMs), e.g., creating net new voice, video, or images
However, across almost all use cases at these companies, it’s clear that an intentional approach to the effort sets the stage for sustained impact. The VA is engaging with our portfolio companies by providing them with a 3-stage framework for their experimentation with AI: Focus on the Foundation, Prepare with Intention, and Iterate and Scale. This allows portfolio company teams to establish a proper data and governance foundation, choose the right process and manage associated risk, and scale via team capability-building and small wins that increase momentum.
Three Stage Approach to Effective AI/GenAI Deployment
Stage 1: Focus on Foundation
Ensure data readiness: Before automating processes, we believe it’s important to ensure that data foundation and quality are in place. Since GenAI can surface data from any system, solidifying the data also quickly highlights any inaccuracies or incongruencies in data across systems.
Establish governance parameters: The best tactic is to integrate GenAI as a value creation accelerator within an existing initiative. If AI is run as a separate program that must be prioritized against a myriad of existing priorities in the company, it loses steam and can easily become subsumed by other concerns.
Stage 1 case study on data readiness:
One portfolio company is a leading provider of modular space solutions for sales and rentals in Northern Europe. The company wishes to increase the proportion of larger and longer-term contracts. By understanding how and when the contracts are renewed, fleet resources can be better allocated and bid pricing can be more competitive. Current pain points exist in data ownership, data modeling, and data accessibility. The VA team is using AI with the company to analyze its historical contract data to predict the probability and length of extension for existing open and new contracts. The work requires onboarding legacy data into the current data warehouse to enrich the available data, as well as uplifting the data processes to create ‘ready-for-AI’ datasets for each data asset.
The projected commercial impact will be an increase in fleet utilization through more accurate forecasting of contract length and module return. An additional objective is to increase bid competitiveness, since a more accurate prediction for contract length at the beginning of the contract will feed into the pricing model.
Stage 2: Prepare with Intention
Select enablement process carefully: Before starting on the AI journey, it is crucial for companies to prioritize the process to be automated based on business value. Once identified, re-imagine the process from an automation lens and detail how each change will drive accretive impact.
Manage risk: The biggest way to mitigate potential reputational or compliance risks of erroneous GenAI output is to maintain a “human-in-the-loop” (at a minimum during the model’s training period and ideally throughout the process redesign).
Revisit the build vs. buy decision: The power and speed of AI development, coupled with an ever-evolving SaaS vendor/capability landscape, render the “buy vs. build” conundrum even tougher for companies. Large incumbent IT vendors and new startups alike are embedding AI to build differentiated solutions. Both the one-time and recurring costs of building and maintaining a customized, bottom-up AI solution remain high. To address this, we believe that companies should first optimize the process and carefully evaluate all implementation options (even non-AI options like robotic process automation) before making the final call. Mid-sized portfolio companies typically benefit from leveraging AI features from their existing vendors, and/or buying off-the-shelf tools with necessary functionality instead of trying to build custom solutions.
Stage 2 case study on process selection:
One of our portfolio companies is a Canada-based benefits provider. For customer claims that cannot be automatically adjudicated or answered, humans are typically involved for verification, and claim handling can take up to 10 minutes to locate the correct policy documentation. Working with the VA, the company built a proof-of-concept agent-facing conversational GenAI platform that answers customer policy inquiries. 25% efficiency has been achieved thus far, due to an 80-90% reduction in time to look up and action customer policy information.
To select this customer engagement scenario, the team conducted a broad AI opportunity scan and identified 15 potential use cases in various areas across the organization. They subsequently narrowed down the list by data readiness, complexity of the overall process, time to value, and presumed ROI. After additional conversations with key business leaders and stakeholders, the team chose a customer engagement opportunity. They scoped the project to be narrow enough to contain risk yet general enough to be applicable to other insurance plans and teams in the customer support group.
Stage 3: Iterate and Scale
Build momentum via small wins: It’s critical to choose a modular enough process and granular enough use case such that GenAI-spurred automation can occur relatively quickly (within 6-10 weeks). A succession of small wins within the use case then serves to both demonstrate success and facilitate momentum for AI in the organization. We think that unveiling the roadmap little by little eases the transition and clarifies the value added at each step.
Enhance team capabilities: Companies should consider whether to build capability in-house or to collaborate with a trusted partner. Upskilling should then continue at regular intervals to ensure maximal value is derived from the GenAI implementation on an ongoing basis.
Stage 3 case study on incremental wins:
One portfolio company is a global digital technology services & platform solutions provider with thousands of developers. The company leveraged an AI-driven developer productivity tool to accelerate coding, code review, and test generation by proposing code options based on text prompting of the development objective. They first piloted the tool with a small group and extended a scale-up roadmap from there. The pilot scope included baselining the current developer productivity, introducing the productivity aid with proper training, and measuring adoption and the delta in productivity. To date, developer productivity has improved over 15% across the entire development base, and the company anticipates further gains in developer experience and code quality. Continual small wins based on an initial proof of concept pave the way for ongoing productivity gains and significant engineering improvements.
Conclusion
As VA experts support our portfolio company management teams across their development of various AI/GenAI use cases for their business’, a three-stage framework for optimal deployment has emerged. Adherence to this framework and the attendant lessons learned will help our portfolio decide where to focus and how to unlock robust value from their engagement with this powerful technology.
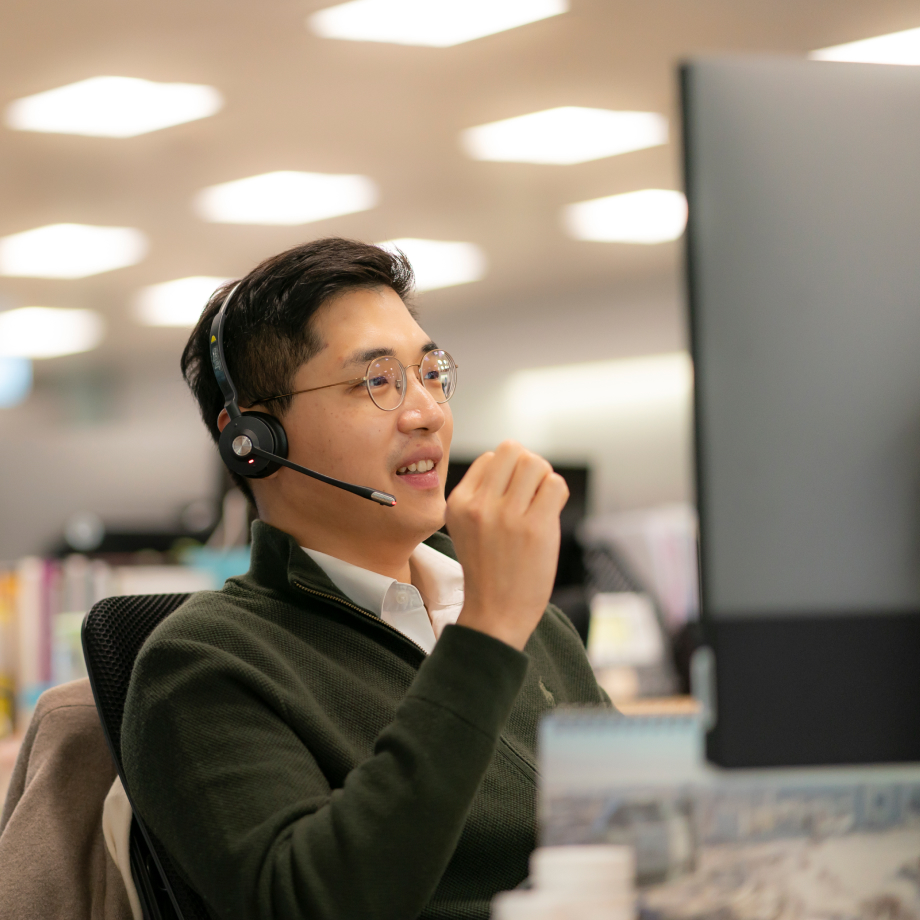