Harnessing the Power of AI to Enhance Investment Decision-Making
The goal of active, data-driven investment strategies is to achieve an informational advantage – knowing more, seeing more clearly and acting faster than other market participants. This involves identifying, interpreting, and acting on key information before it becomes fully priced into the market. In assessing the quality and valuation of companies, data-driven investors historically relied largely on quantitative and structured information, such as a company’s price-to-earnings ratio, along with market data such as stock performance and trading volume. This list has grown in recent years with the availability of structured datasets such as data on shipments, internet web traffic and credit-card transactions.
While these metrics remain important, the investment decision-making process has evolved significantly in recent years, a process that has accelerated with the rise of artificial intelligence (AI). Data-driven investors now have access to larger, less structured, and more complex datasets than ever before. Non-traditional sources such as news articles, regulatory and patent filings, and transcripts and audio recordings of earnings calls require new methods for analyzing data and extracting actionable insights. The progress of AI has provided investors with increasingly powerful tools for processing these more nuanced and unconventional datasets with the goal of constructing alpha signals and improving decision-making.
Leveraging AI effectively involves more than just the necessary technology, such as scalable storage and clusters of central and graphics processing units (CPUs and GPUs). An AI-driven investment process requires a well-designed strategy for acquiring data with the potential to yield meaningful insights, cleaning it and incorporating it into an integrated framework. In other words, we think an impactful and scalable AI strategy is built on a solid data strategy that is embedded in a company’s culture and philosophy.
The rise of AI technology has not diminished the importance of the people who use it. In fact, the opposite is true. Harnessing the full power of big data and AI requires data scientists capable of formulating high-quality hypotheses and driving novel ideas. They need to know which methods and techniques will deliver the most differentiated insights from the data available, and how to use these insights to accomplish specific tasks efficiently.
Both these elements – a robust data strategy and innovative data scientists – are essential to power an active, data-driven investment strategy, in our view. In this article, we draw on the experience of Goldman Sachs Asset Management’s Quantitative Investment Strategies (QIS) team to explore these two elements and the solutions they seek to make possible.
Strategy and Science
When deployed effectively, AI can allow investors to extract meaningful insights from complex and unstructured datasets that were previously incredibly difficult to process. The foundation of this process is data.
We believe investors need a comprehensive strategy for acquiring and processing datasets with the greatest potential to yield meaningful insights. This strategy should cover areas including data quality, data infrastructure, governance, testing, deployment and oversight. The other critical component is a skilled workforce of data scientists.
Data scientists with experience working at the intersection of data and AI are better prepared to select appropriate models and identify and mitigate biases that may be inherent in available datasets. If these biases go undetected, they can become exaggerated in the training of an AI model. When considering AI tools, data scientists need to evaluate the trade-offs between complexity, transparency, and interpretability. By understanding the strengths and limitations of AI techniques, experienced data scientists can help ensure that the datasets and models chosen for a specific project are aligned with its objectives.
It is not sufficient to ask a generative AI model to predict stock returns or recommend stocks to buy or sell. We believe AI techniques should be viewed as tools to test and capture hypotheses during investment analysis. The true value lies in humans’ ability to generate high-quality hypotheses and innovative ideas that can be applied to a dynamic set of data and potentially provide a competitive edge over the long term.
Analyzing the Audio
One example of a productive hypothesis with the potential to leverage the efficient use of AI models is our QIS team’s belief that the sentiment expressed by corporate officers and managers on earnings calls can provide insight into companies’ potential future performance. These individuals typically have a strong understanding of their companies’ prospects, and their behavior and remarks may hint at shifts in their thinking.
For more than a decade, the QIS team has validated and implemented this hypothesis as an investment strategy using a variety of techniques. By leveraging Natural Language Processing tools such as Bidirectional Encoder Representations from Transformers (BERT)1 to process earnings call transcripts, the QIS team can evaluate the textual sentiment of what management is saying. Since 2023, we have complemented this by expanding our focus to include how they say it.
The data for this analysis can be derived from audio recordings of earnings calls. Audio-based analysis allows us to capture emotional nuances in the delivery of a message, shifts in tone and emphasis, that would be difficult to discern from a transcript. We find that the question-and-answer portions of earnings calls contain a high level of this additional information because of the immediacy of the exchanges and their unscripted nature. Deep learning2 techniques can be applied to raw earnings audio of corporate officers and managers to extract a wide spectrum of features to analyze their delivery against indicators such as ambiguity, tonal emotion and evasiveness. While human analysts could perform a similar task, the use of AI allows investors to process audio from thousands of calls efficiently and control the possibility of human biases. Our QIS team, for example, has access to more than 400,000 hours of audio data from earnings calls.
Audio recordings present challenges that do not occur in textual analysis. They often contain periods of silence, background noise and music. Some speakers are more expressive than others. Speakers can have different accents or be speaking a language that is not their mother tongue. Because of this complexity, our QIS team relies on raw data which we clean ourselves to preserve potentially significant nuances. We analyze a speaker's delivery against their own vocal profile to provide a consistent baseline for comparison. The output of the deep learning models can then be used to help form a more robust alpha signal.
Lead-Lag Effects
Another example of a productive hypothesis is that lead-lag relationships between stocks can arise indirectly through exposure to common themes. If we can quickly identify these market themes at scale, as well as a given stock’s exposure to them, we may be able to capitalize on emerging trends.
Natural Language Processing techniques such as embedding models allow us to detect these themes systematically in a variety of textual datasets including patent filings, company press releases, news reports, and sell-side analyst reports.3 Once these themes are identified, we can track the performance of the companies to gauge and capitalize on momentum in these various thematic trends. The QIS team currently leverages 290,000 analyst reports, 2 million news articles, and 50 million patents globally per year.
Take news reports, for example. Our QIS team thinks companies that are frequently mentioned in the same news stories are likely to be economically linked, and that investor underreaction to these links can create lead-lag effects in stock returns. Based on this hypothesis, AI techniques can be used to analyze the large volume of news we monitor daily. This allows us to build a linkage web across thousands of stocks and observe the ripple effects resulting from market events, which in our view can impact equity returns.
While traditional methods of analysis could be overwhelmed by the ceaseless flow of new information, a quantitative, data-driven approach powered by AI models allows us to understand how a global event could potentially trigger first- and second-order effects. A disruption in natural-gas supplies could push up the price of fertilizer, for example, contributing ultimately to an increase in global food prices. In a case like this, our models could identify the companies within this linkage web that would be impacted.
A Comprehensive View
The rapid evolution of AI techniques has provided investors with powerful new tools for evaluating firms and managing risks. In addition to traditional data such as financial statements, investors can now draw on vast, complex and less structured datasets that can provide insights into additional influences on a company’s future value. This process begins and ends with a human in the driving seat. It starts with a strategy for acquiring, cleaning and processing data, and relies on data scientists to provide the novel hypotheses that maximize the effectiveness of AI techniques, as well as to continually monitor, innovate and finally to interpret and draw out insights. By harnessing the power of AI, investors can achieve a more comprehensive understanding of companies and improve their decision-making and risk-management processes.
1 BERT is a deep learning language model that can be fine-tuned to determine the likelihood that an input is a positive, neutral or negative statement.
2 IBM. Deep learning is a type of machine learning that uses multilayered artificial neutral networks to simulate the decision-making process of the human brain. As of November 26, 2024.
3 Embedding models are used to convert data into a numerical format for processing by computers. For example, they can take words and sentences and represent them as vectors, which are lists of numbers. These vectors capture the meaning and relationships between data points. Words with similar meanings will have similar vector representations.
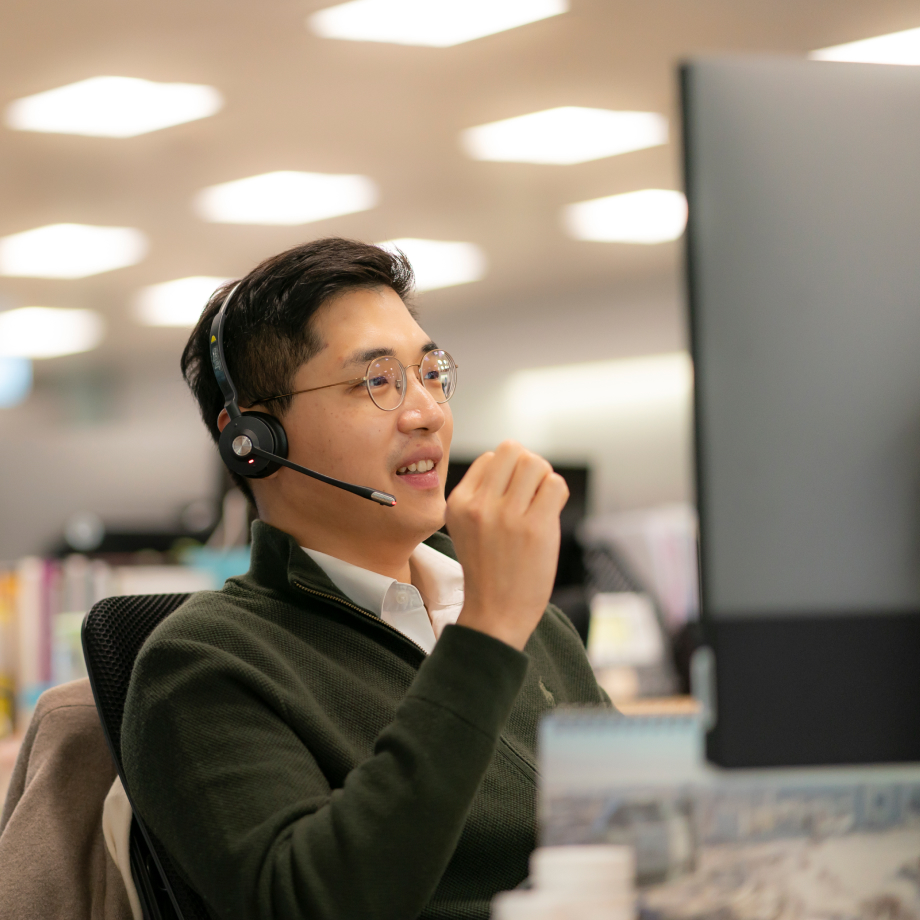