Building Foundations: Speed Versus Strategy In The Race For AI Adoption
GenAI holds transformative potential, and we believe successful adoption by corporate management teams across sectors is likely to contribute to long-term company success. But like any tool, capitalizing on GenAI’s capabilities requires clear understanding of its value levers and complexities. In our view, corporate leaders should consider ways to holistically integrate GenAI into firm-wide strategies. Management teams should evaluate ways the technology can be used as tool for true strategic differentiation, not just efficiency gains. Learning curves are likely to be steep for executives in industries that have historically had less experience with technological innovation. However, we see ways for a wide range of businesses to ensure the right foundations are in place to harness GenAI’s game-changing potential.
Evaluating The Power of a GenAI Adoption Strategy
GenAI adoption approaches will ultimately vary at the firm level but thinking through fundamental questions can be a helpful starting point to stay competitive, add value, and avoid potential setbacks. We think there are four key questions corporate management teams should seek to address.
How can GenAI support our unique customer value proposition?
While GenAI may significantly boost customer experience, automation, and even fraud detection, not all uses of the technology will be equally impactful. For instance, if customer value is fundamentally derived from physical products or interactions, GenAI may be unable to recreate core features and services, making it less effective and therefore a lower priority for certain businesses. Excessive reliance on GenAI for customer interactions could result in a loss of personal connection, harming loyalty, and brand reputation. The key lies in identifying synergies between human expertise and the capabilities of GenAI. Companies that struggle to strike the right balance between automation and human touch may suffer.
How can we harness GenAI to distinguish ourselves from our competitors?
Novel technologies can quickly become a standard part of doing business, while subsequent innovations change the competitive landscape over time. The rapid rise and evolution of GenAI suggests that first-mover advantages disappear quickly. Companies are likely to require an especially high level of adaptability to stay competitive. As such, it is important to understand how GenAI monetization models and paths to value creation could be disrupted, and whether GenAI enables new entrants to rapidly replicate core customer propositions. For instance, GenAI-driven efficiency gains may be competed away by the emergence of low-cost, potentially AI-native solutions. Lower switching costs enabled by simplified onboarding and migration processes may threaten incumbents relying on lock-in effects.
Not all businesses need the same degree of analytical power and accuracy to drive value proposition and maintain their competitive edge. This has implications both for the data input requirements for GenAI models, and for output oversight. For example, a high degree of accuracy is sufficient for a GenAI-generated product pitch. Other applications, like interpreting a medical image or providing legal advice, require zero error tolerance. As the level of accuracy required increases, so too does the need to train GenAI models on a high volume of data. In such cases, the accuracy and speed of analysis is greater, but the need for human oversight remains until a greater level of trust has been achieved.
Efficient GenAI deployment will hinge on data readiness, quality, integration, security, and privacy. We believe that ownership of proprietary data will be the key factor to create the strongest AI moat. Unique data will produce unique products and services, while companies applying similar technology to similar data will likely yield undifferentiated results and fall into a creativity gap. However, there is also the risk of a company’s model unintentionally helping competitors (i.e., private or proprietary data can be used to improve models that will be used by competitors). At the same time, sophisticated data management programs to ensure the security of proprietary and potentially regulated data may mean higher ongoing maintenance expenses.
What is the all-in cost of AI implementation, and does our company have the resources to bear this cost?
The primary, direct costs of AI implementation are computational power and data costs. The type and level of AI that a company will incorporate—and therefore the spend—will depend on the answers to the first two questions above. For instance, if corporate strategy calls for developing and maintaining proprietary large language models, the associated costs can be extremely high.
Building and training an AI model can be expensive and time-consuming. Costs include hardware, engineering, data acquisition, testing and computing. However, unlike in earlier computing booms, these costs may not decline meaningfully once the models are trained, since running trained models still requires large amounts of computing power to perform the billions of calculations necessary to respond to each prompt. Because of this, some companies are shifting toward running smaller models (while still being trained on larger amounts of data). On the other hand, open-source models can be free (albeit with potentially limited maintenance and support). A number of technology businesses are expanding the ecosystem to offer more, better and cheaper AI solutions to their customers for a variety of use cases.
Whether the models are large or small, the costs of infrastructure to support GenAI are a key budget factor. Without the infrastructure to support increasing demand for computing capacity, AI transformation will not be possible. Data centers provide the infrastructure necessary to train and deploy complex algorithms, and machine learning (ML) and large language models are already driving increased demand for data center capacity, with approximately 20% of demand across the data center landscape expected to come from AI-related activities by the end of the decade.1 Furthermore, AI will substantially increase the amount of power required to operate the data centers. For instance, a single ChatGPT search consumes 6-10x the power as a traditional Google search2 Recent studies forecast a 15% compound annual growth rate (CAGR) in data center power demand from 2023-2030, driving data centers to make up 8% of total US power demand by 2030 from about 3% currently3 In many cases, the meaningful increase in power consumption will require improvements to the existing power grid and buildout of new energy generation capacity.
Given the need for adequate access and long queues to connect to the power grid, competition and the physical constraints of infrastructure will likely drive up costs. Data centers and power generation assets can take some time to build, and strategic data center locations are finite. Currently, AI operators are struggling to find capacity to deploy current AI workloads, which is resulting in data center owners and operators having strong pricing power for a key cost component. While this trend is currently US focused, it is expected to become global over the medium to long term.
Talent costs are also rising as high demand for AI talent meets a limited supply of workers with expertise in the relatively new fields of processing and robotic process automation, deep and ML and natural language processing. Secondary costs include retraining, organizational change management, and legal—all of which could have significant impact on business plans that do not manage GenAI deployment wisely.
Are we properly accounting for societal concerns and their potential impact on AI adoption?
This question may seem to be beyond the scope of any individual company to address. Nonetheless, the three concerns outlined below will impact the future of AI use overall and should therefore be kept in mind by anyone investing in this area.
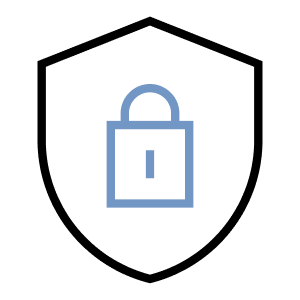
Security risks are a paramount concern given the possibility of cyberattacks that can manipulate underlying data in the models, changing the output and compromising model integrity in ways that are difficult to detect.
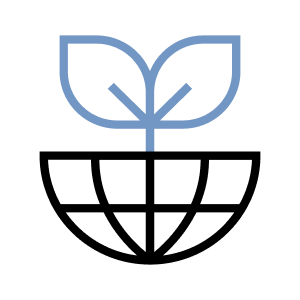
These concerns stem from both the energy consumption required to power the models and from high demand for raw materials needed to build and replace the associated hardware (with fast replacement cycles due to rapid technological advancement).
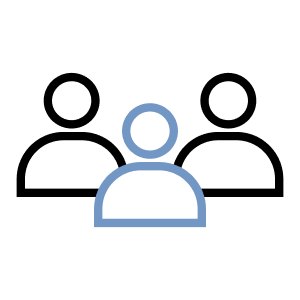
Societal impact concerns stem from the lack of transparency and interpretability of AI-generated outputs, raising questions around accountability and potential biases in the data, especially in critical applications where human lives or rights may be at stake. Misinformation, deepfakes, and various attempts to influence human behaviour can erode trust in institutions, exacerbate societal divisions, and potentially disrupt democratic processes.
These concerns may have commercial implications, potentially impacting the wider adoption of the technology. What if these concerns make the public more reluctant to accept GenAI as the key means of interaction with some companies and experiences? Can underlying data owners sue the model owners for breach of copyright? Could major real-world events (e.g., AI-driven industrial accidents), online events (e.g., targeted disinformation and rise of deepfakes) in combination with social and ecological concerns lead to a tipping point?
Human oversight and hybrid use of technology and human judgement will be critically important for managing the societal challenges and their commercial impacts. We believe governance structures can become a key competitive differentiator for companies. Accountability will be critical in earning and keeping customer trust, and companies that create transparent and explainable GenAI systems, set clear guidelines, and earn trust around safety and privacy will likely have a long-term advantage.
At the level of individual companies, how these issues are addressed can itself be a tool for strategic differentiation. Companies can deploy GenAI in ways that address their customers’ concerns, with a view towards their unique value proposition and risks as well as prioritizing their customers’ key focus areas. Considering the complexity and pace of this technology’s adoption, efforts by companies to build and maintain the trust of their customer base through transparency and clear communication could become a key differentiator.
Looking ahead
When introducing GenAI, it is important to build the foundations in terms of data sophistication and readiness, technical infrastructure, talent, organization and governance today, in order to harness the evolving benefits of this fast-paced technology in the future.
It is also important to understand the ways in which GenAI is different to traditional AI/ML. AI/ML has not done a great job of reasoning and content generation, and creativity has been the holy grail. But GenAI is becoming strong in both areas, enabling previously unimagined levels of creativity. It is possible that over time, GenAI will not only answer our questions accurately, but also tell us what the question should have been. This can lead to a profound paradigm change across industries.
Staying ahead of the curve will be critical—even the best product and company doesn’t have a second to be complacent in this fast-paced environment. As we previously highlighted, technology as a standalone thesis will not be sufficient to drive returns. Even more than speed, we believe the long-term winners and losers in this race will be defined by the strategy with which they adopt this new technology. By asking the questions outlined above, management can gain the unique company-specific insights needed to effectively integrate GenAI in a way that drives tangible transformative value.
1. Goldman Sachs Global Investment Research. “Generational growth, AI, data centers and the coming US power demand surge.” As of April 2024
2. Goldman Sachs Global Investment Research. “Generational growth, AI, data centers and the coming US power demand surge.” As of April 2024
3. Goldman Sachs Global Investment Research. “Generational growth, AI, data centers and the coming US power demand surge.” As of April 2024
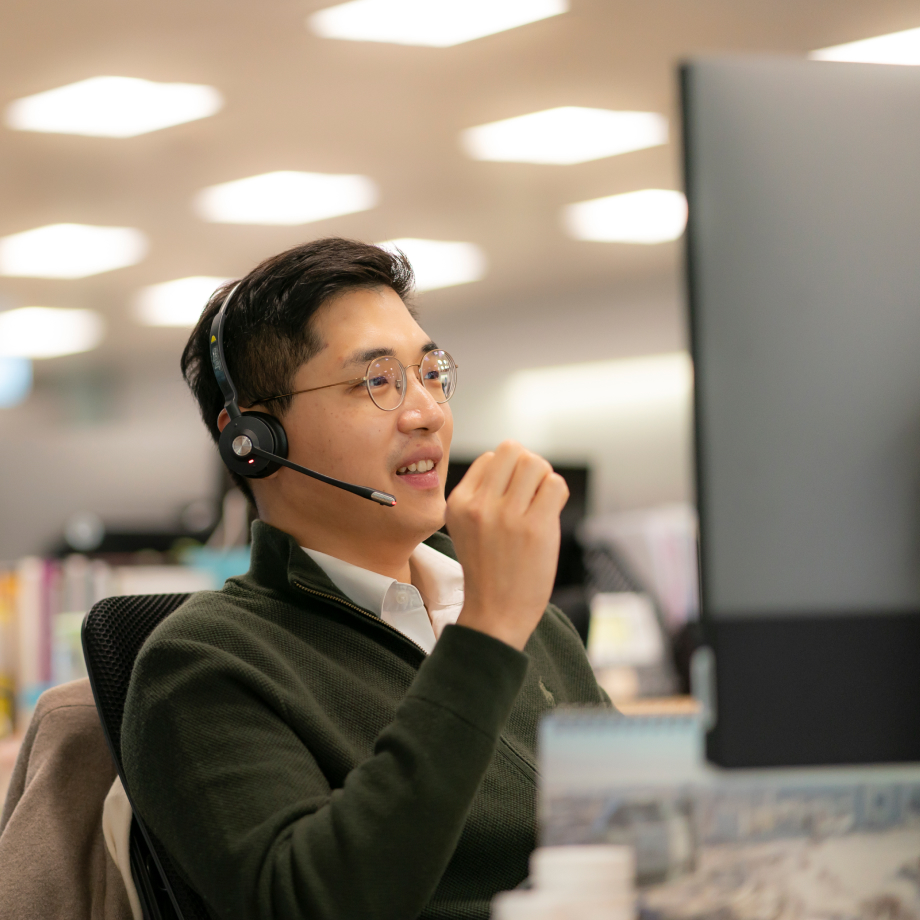